“SCOUT” helps researchers find, quantify significant differences among organoids
Unbiased, high-throughput analysis pipeline improves utility of “minibrains” for understanding development and diseases such as Zika infection.
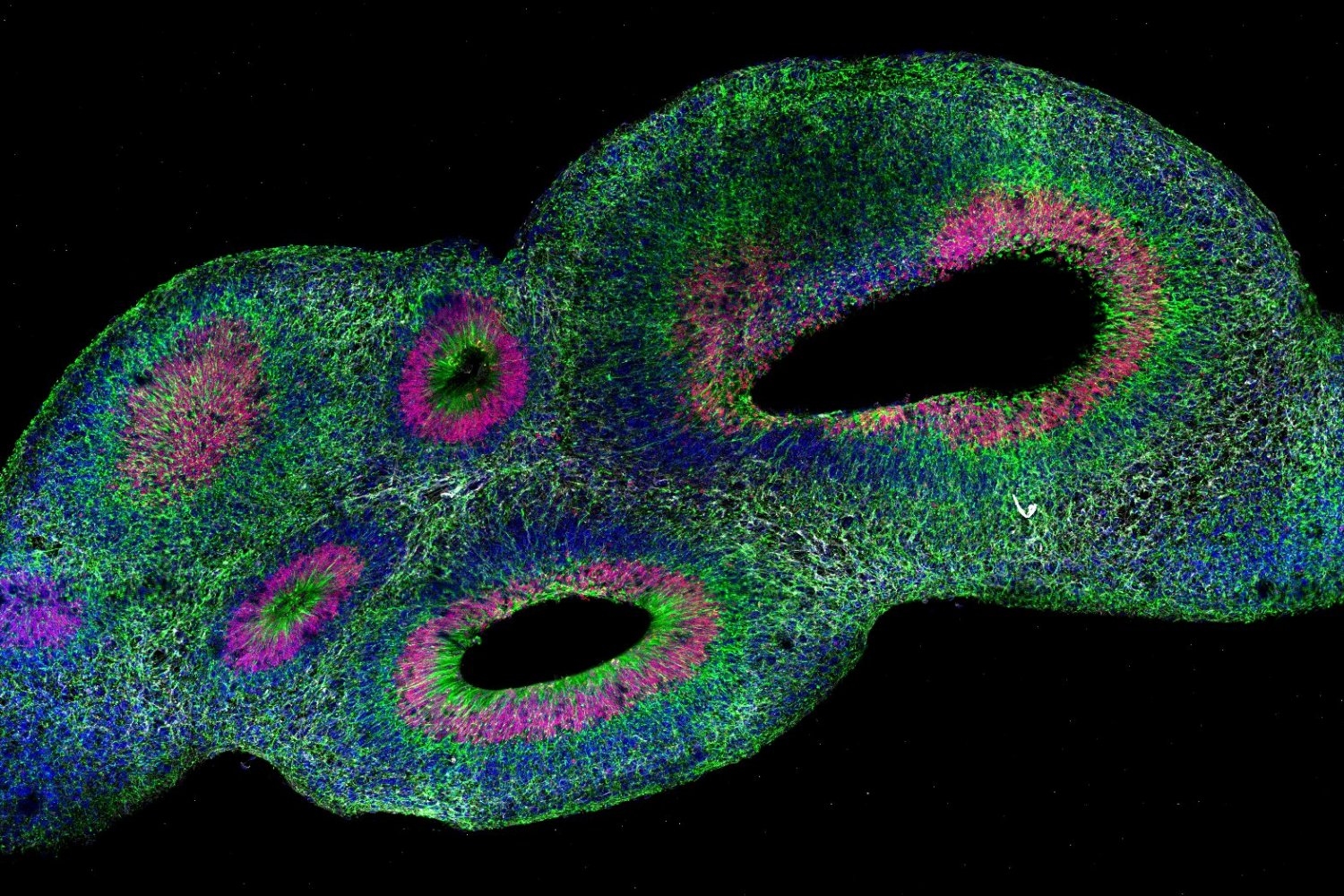
The ability to culture cerebral organoids, or “minibrains,” using stem cells derived from people has given scientists experimentally manipulable models of human neurological development and disease, but not without confounding challenges. No two organoids are alike and none of them resemble actual brains. This “snowflake” problem has held back the science by making scientifically meaningful quantitative comparisons difficult to achieve. To help researchers overcome those limitations, MIT neuroscientists and engineers have developed a new pipeline for clearing, labeling, 3D imaging, and rigorously analyzing organoids.
Called “SCOUT” for “Single-Cell and Cytoarchitecture analysis of Organoids using Unbiased Techniques,” the process can extract comparable features among whole organoids despite their uniqueness — a capability the researchers demonstrate via three case studies in their new paper in Scientific Reports. In one of the case studies, for example, the team reports new patterns of disruption in organoid development from Zika virus infection, providing new insights into why babies born to infected mothers can exhibit severe neurological deficits.
“When you are dealing with natural tissues you can always subdivide them using a standard tissue atlas, so it is easy to compare apples to apples,” says study co-lead author Alexandre Albanese, a research scientist in the lab of the paper’s senior author, Associate Professor Kwanghun Chung. “But when every organoid is a snowflake and has its own unique combination of features, how do you know when the variability you observe is because of model itself, rather than the biological question you are trying to answer? We were interested in cutting through the noise of the system to make quantitative comparisons.”
Albanese co-led the research with former MIT chemical engineering graduate student Justin Swaney. The team has taken the added step of sharing their software and protocols on GitHub so that it can be freely adopted. Chung says that by sharing many of his lab’s tissue processing, labeling, and analysis innovations, he hopes to speed up biomedical progress.
“We are developing all these technologies to enable more holistic understanding of complex biological systems, which is essential to accelerate the pace of discovery and the development of therapeutic strategies,” says Chung, an investigator in The Picower Institute for Learning and Memory and the Institute for Medical Engineering and Science as well as a faculty member in the departments of Chemical Engineering and Brain and Cognitive Sciences. “Disseminating these technologies is as important as developing them to make a real-world impact.”
Abstracting architecture
Several of the Chung lab’s technologies are components of the SCOUT pipeline. The process starts by making organoids optically transparent so they can be imaged with their 3D structure intact — a key capability, Chung says, for studying whole organoids as developing systems. The next SCOUT step is to infuse the cleared organoids with antibody labels targeting specific proteins to highlight cellular identity and activity. With organoids cleared and labeled, Chung’s team images them with a light-sheet microscope to gather a full picture of the whole organoid at single-cell resolution. In total, each organoid produces about 150 GB of data for automated analysis by SCOUT’s software, principally coded by Swaney.
The high-throughput process allows for many organoids to be processed, ensuring that research teams can include many specimens in their experiments.
The team chose its antibody labels strategically, Albanese says. With a goal of discerning cell patterns arising during organoid development, the team decided to label proteins specific to early neurons (TBR1) and radial glial progenitor cells (SOX2) because their organization impacts downstream development of the cortex. The team imbued SCOUT with algorithms to accurately identify every distinct cell within each organoid.
From there, SCOUT could start to recognize common architectural patterns, such as identifying locations where similar cells cluster or areas of greater diversity, as well as how close or far different cell populations were from ventricles, or hollow spaces. In developing brains and organoids alike, cells organize around ventricles and then migrate out radially. With the aid of artificial intelligence-based methods, SCOUT was able to track patterns of different cell populations outward from each ventricle. Working with the system, the team therefore could identify similarities and differences in the cell configurations, or cytoarchitectures, across each organoid.
Ultimately, the researchers were able to build a set of nearly 300 features on which organoids could be compared, ranging from the single-cell to whole-tissue level. Chung says that with further analysis and different molecular label choices even more features could be developed. Notably, the features extracted by SCOUT are unbiased, because they are products of the software’s analysis, rather than preordained hypotheses about what is “supposed to be” meaningful.
Scientific comparisons
With the analytical pipeline set, the team put it to the test. In one case study they used it to discern trends of organoid development by comparing specimens of different ages. SCOUT highlighted dozens of significant differences not only in overall growth, but also changes in the proportions of cell types, differences in layering, and other changes in tissue architecture consistent with maturation.
In another case study they compared different methods of culturing organoids. Harvard University co-authors Paola Arlotta and Silvia Velasco have developed a method that, according to single-cell RNA sequencing analysis, produces more consistent organoids than other protocols. The team used SCOUT to compare them with conventionally produced organoids to assess their consistency at the tissue scale. They found that the “Velasco” organoids show improved consistency in their architectures, but still show some variance.
Zika insights
The third case study involving Zika not only proved the utility of SCOUT in detecting major changes, but also led to the discovery of rare events. Chung’s group collaborated with virus expert Lee Gehrke, the Hermann L.F. von Helmholtz Professor in IMES, to determine how Zika infection changed organoid development. SCOUT spotted 22 major differences between infected and uninfected organoids, including some that had not been documented before.
“Overall this analysis provided a first-of-its kind comprehensive quantification of Zika-mediated pathology including loss of cells, reduction of ventricles, and overall tissue reorganization,” the authors wrote. “We were able to characterize the spatial context of rare cells and distinguish group-specific differences in cytoarchitectures. Infection phenotype reduced organoid size, ventricle growth, and the expansion of SOX2 and TBR1 cells. Given our observation that SOX2 cell counts correlate with multiscale tissue features, it is expected that Zika-related loss of neural progenitors decreased in the complexity of tissue topography and cell patterning.”
Chung says his lab is also collaborating with colleagues studying autism-like disorders to learn more about how development may differ.
In addition to the authors mentioned above, the paper’s co-authors are Dae Hee Yun, Nicholas B Evans, Jenna M. Antonucci, and Chang Ho Sohn.
The research was supported by funding sources including the JPB Foundation, the NCSOFT Cultural Foundation, and the National Institutes of Health.
What's Your Reaction?
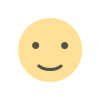
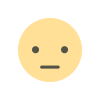
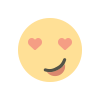
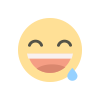
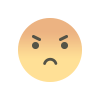
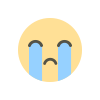
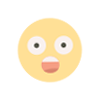